Peter Bailis is the co-founder and CEO of Sisu which is a software that empowers people to make better decisions with data. The company has raised $66 million from top tier investors including Andreessen Horowitz, New Enterprise Associates, and Green Bay Ventures.
In this episode you will learn:
- The future of business
- The importance of data
- How he chooses investors
- How to make pivots in your professional career

SUBSCRIBE ON:
For a winning deck, take a look at the pitch deck template created by Silicon Valley legend, Peter Thiel (see it here) that I recently covered. Thiel was the first angel investor in Facebook with a $500K check that turned into more than $1 billion in cash.
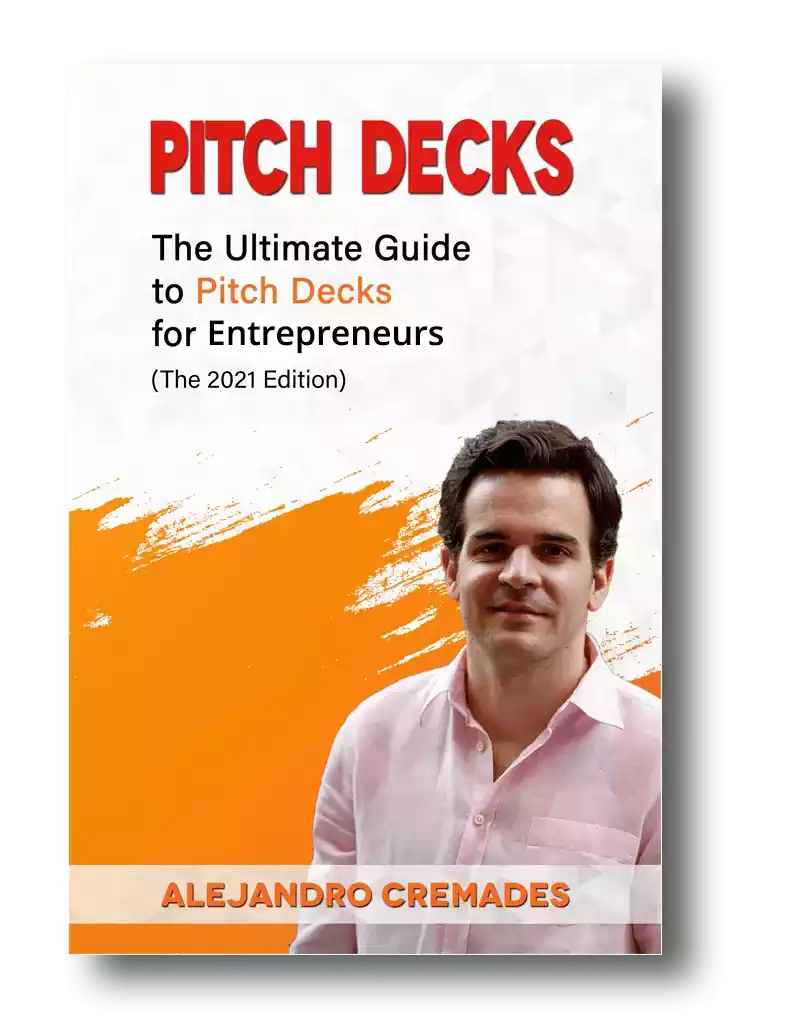
*FREE DOWNLOAD*
The Ultimate Guide To Pitch Decks
Remember to unlock for free the pitch deck template that is being used by founders around the world to raise millions below.
About Peter Bailis:
Peter Bailis is the co-founder and CEO of Sisu which is a software that empowers people to make better decisions with data.
The company has raised $66 million from top tier investors including Andreessen Horowitz, New Enterprise Associates, and Green Bay Ventures.
Prior to this, Peter Bailis was an assistant professor of computer science at Stanford University. Peter Bailis research in the Future Data Systems group and DAWN project focused on the design and implementation of post database data-intensive systems.
Peter Bailis is the recipient of the ACM SIGMOD Jim Gray Doctoral Dissertation Award, an NSF Graduate Research Fellowship, a Berkeley Fellowship for Graduate Study, best-of-conference citations for research appearing in both SIGMOD and VLDB, and the CRA Outstanding Undergraduate Researcher Award.
Peter Bailis holds a PhD from UC Berkeley and an AB from Harvard College, both in computer science.
Connect with Peter Bailis:
* * *
FULL TRANSCRIPTION OF THE INTERVIEW:
Alejandro: Hello, everyone and welcome to the DealMakers show. Today we have a very interesting founder. A founder that left academia to execute on what he thought was an interesting solution to bring to market. It involves data, and there are interesting financing rounds that they have done. Without further ado, I’d like to welcome our guest today. Peter Bailis, welcome to the show.
Peter Bailis: Thank you. I’m excited to be here.
Alejandro: Peter, you were born and raised in Omaha, Nebraska. How was life growing up there?
Peter Bailis: Omaha’s a great place to grow up. There’s not a lot that goes on in Omaha, relative to some of the bigger cities in the states, but there’s a lot of space, lots of places to go, do things like light off model rockets, and lots of basements to play music in. On the whole, pretty good. I always knew there was a bigger world out there. But it was nice to grow up somewhere where it felt calm, you could do whatever you wanted, and people were nice and friendly.
Alejandro: And the place where Warren Buffett is. Right?
Peter Bailis: Exactly. So Warren Buffett is probably the most famous. There’s a big Indie rock scene in the early 2000s that sort of petered out. So those are the two things that in high school, we were best known for. And now, I think it’s just Warren Buffett.
Alejandro: Got it. Being there, I’m sure that life is super comfortable and easy, why did you decide to pack the bags and do computer science in a place like Harvard?
Peter Bailis: That’s a good question. I had a fantastic math teacher in high school who, as part of the curriculum, had us read a bunch of books, research papers, like the book called Chaos, by James Gleick. It talks about how chaos theory formed and how it relates to differential equations. It’s one of the craziest advanced calculus classes I’ve heard of. Not only were a bunch of people in this one book that made a big influence on me on the East Coast, a bunch of folks at MIT, but also, it seemed like there was so much going on in these universities outside of Nebraska. Nebraska’s a great university, simply for what it’s worth, but it seemed like a lot of the action was outside. In terms of computers, I never took a computer science class, but this one math teacher who encouraged us to read about fractals and nanoprocess and stuff. I ended up writing a bunch of Python programs to plot these things in ASKE in my junior year of high school. I thought it was so cool. I had no idea how to write programs, but I was able to build these kinds of mathematical visualizations. Once I got to college, I figured, “I’ll take a CS class,” and the rest is history.
Alejandro: Then you go into this track to be in academia. You went to Berkeley to do your doctorate. Then you go to Stanford. How did you land in Stanford?
Peter Bailis: I had a lot of great mentorship along the way. That’s one big part of it. I’m really fortunate. I’ve always been interested in this intersection between what the theory says should be possible and what people actually want to do with computer systems. It runs a kind of funny phase of computing where a lot of the low-hanging fruit has been picked, like some of the fundamental results in distributive computing, what’s possible, and impossible without reaching agreement on a distributive network. And even some of the stuff in competition complexity — just like a lot of low-hanging fruit has been picked. But in some sense, in academia, a lot of the easy problems are solved. It’s kind of funny. There’s not a lot of fields in academia where they’re like 50 years old and have the impact they do on society — compared, like with math, where you have literally thousands of years of study. But nevertheless, the way in which people continue to apply computing seems always to break the conventional models. My doctoral work was basically looking at forever using sequel databases. Part of the reason they were using them is because they always guaranteed a response. When you deploy them across data centers, they’re very quick to respond. It turned out this is like a very particular network model, which the conventional literature didn’t necessarily apply. We were all like, “Take the old theory and apply it to this new setting.” It turned out you could get computers to do things that historically had never been able to do without communications and coordination presetting. The long story, short, is, a lot of the low-hanging fruit in computer science theory has been picked, but the actual application to real problems and systems in the world continues to be a really fun space to play. People keep finding more and interesting and creative ways to do things with computers that the models don’t exactly lineup. I like that gritty intersection between what the textbooks say and what people are trying to do with systems.
Alejandro: Very cool. How old were you when you landed in Stanford as an assistant professor?
Peter Bailis: I think I was 25 when I accepted the offer and 26 when I showed up.
Alejandro: Wow. So you were probably one of the youngest professors there. Right?
Peter Bailis: Definitely when I started. Yeah. Fortunately, they gave me the benefit of the doubt, but yeah. I think the nice thing thing about academia, especially in the field that moves as fast as CS, there are definitely some really greats in the field like Mike Stonebraker is an amazing database systems researcher. But even guys like Mike have to stay on top of the box; the field moves so fast. I got a great group of students to work with, and we were able to raise funding, and do the research we wanted, and jump off the races. Stanford has been a really supportive place.
Alejandro: Talking about research, you had the research project done. Can you tell us about this research project?
Peter Bailis: Yeah. Our goals were twofold. One was, I love working with other researchers. I think it’s the best part of being in academia is working with other super-smart people. You’ve got a bunch of really great grad students and a bunch of really awesome faculty. So I wanted to figure out, what can we do in everything from data to computer systems to hardware that would make a big impact on a multi-year scale? I partnered up with Matei Zaharia, who had started Spark. He was one of my colleagues at Stanford. Chris Ré, who’s a pioneer in machine learning and machine learning interfaces. Kunle Olukotun, who is a hardware pioneer, built some of the first multi-threaded processors. And we said, “What can we do as a team if we teamed up. So it was a no-brainer to work with some really smart folks and my colleagues. Then the question, “What are we going to work on?” At the time, especially back in 2015, we were still in the heyday of all these machine learning competitions where people were getting better and better accuracy on benchmarks like ImageNet. It seemed like accuracy is the only important metric to optimize for simplifying a little bit. We started to ask the question, what would it mean not to try to make the model more accurate, but what does it mean to make models more usable. You go talk to folks like Brain or Google, or some of the teams at Facebook, it’s certainly clear they have one of the most intense and rich concentrations of PhDs working on some of the hardest problems in computer science at scale in the history of humanity. But the tradeoff is, if you look at these organizations as a whole, even like Think Scale organizations, only a very small fraction of the people inside of these organizations have access to ML, talent, and data science talent. The toolkit, by and large, even for a very profitable large businesses from like Google Play to Facebook Messenger is still in its infancy in terms of making machine learning not just accurate, but usable, the standard software engineering practices around developing, building, shipping, monitoring models still remain in their infancy. We said, “As a team, what can we do full-stack, building out systems for use for machine learning, which is the curdle of this five-year Dawn project.
Alejandro: Very cool. Then what happens next?
Peter Bailis: You let many flowers bloom. Our commitment in Dawn was we would get some of the best forward-looking industrial partners to help fund our research. We had a great slate of industrial sponsors who would subsequently and in return, fund open-source software development in this space of usable machine learning. We had work that continues this day on new interfaces for collecting large amounts of training data. There’s a project called Snorkle, had seen really wide adoption, including Google. They wrote some papers recently improving the quality of Google ads but also working with folks in the med school and so on. We had worked on improving the performance of data science pipelines, and you could pilot efforts, this project called Weld. My work and where a lot of my time and energy went was looking at the problem of structured data. The most valuable data in the world, despite what you might expect from deep learning, is not in images or in text, but it’s in giant tables and warehouses like Snowflake, Redshift, and BigQuery. A large fraction of my work as part of Dawn was how do you enable everyday line of business users who are tracking metrics like engagement and activation and churn, using on top of the structure data, how can we use this data more effectively and efficiently? I spent a bunch of time working on that, and that ultimately took me to Sisu.
Alejandro: And Sisu is the business that you’re running now. I know that for you, one of your biggest decisions was leaving academia. Right? So, here you are at one of the best universities in the world. For me, when it comes to entrepreneurship, it is my number one, the one that I would definitely pick number one. But here you are, one of the youngest fellows there. The students could be even older than you, and all of a sudden, everything takes a different course here. You literally make the decision to leave academia behind and go at building and scaling your own business. Tell us, when does Sisu really become tangible for you and the option that you said, “I need to pay more attention to this, and perhaps this is what’s going to be my next phase.
Peter Bailis: The way that Stanford works is, they give every faculty member two years to go on leave out of every seven to work on whatever you want. A bunch of famous folks you’ve probably heard of: Andrew Ings, Daphne Koller, Sebastian Cheung. It’s a pretty well-trod path to take this time off and go and build something. I think what you’ve seen increasing in recent years is much more common-model where you’ll partner with a business operator and use your day a week of consulting time to go and build a company that way. For me, long story short, I felt like there was such a compelling need in the market, and every from business model to go to market, packaging, technology, wasn’t just going to be a plug-n-play, better, faster, cheaper business model where we’d easily drop in a business operator. I said on my death bed. When I look back, do I want to have done the safe thing of spending 20% of my time on this thing, or do I want to go two feet in and build a company from scratch? For me, the itch was big enough there that I had to go and do it. For context, here, what we had built at Stanford was a set of technologies that would enable line-of-business owners, like your product manager — I’ll give some concrete examples. The product managers who ran the Skype teams at Microsoft get all this data about calls placed worldwide. They look at metrics like how many calls are placed today versus yesterday, or the quality of service now versus in the past, and there are literally hundreds of different factors that can influence quality of service like what phone was the call placed on, what was the application version, what’s the ISP, and so on. These factors are always changing. We came up with new techniques and algorithms for basically finding the root causes and the key drivers behind metrics like quality of service. Hypothetically, you might say given ISP role that had changed with network configuration, and as a result, Android, Galaxy, S10 devices are experiencing lower than usual quality of service. You would never have found that in a million years if you spent the time manually looking at this. We had built prototypes that let these users, including some of the folks at the Skype team. Microsoft was a big partner in the early days of our research. They helped people answer the question of why. Why are my metrics changing? I’m happy to go into more detail here, but we essentially found that if we could do this at Microsoft scale or Facebook scale. We were at papers with Google about similar techniques to answer this question of why are my key metrics changing? It worked for these FANG-scale companies. There was very likely a massive set of users who could also benefit from this technology that we necessarily wouldn’t reach just by doing more research on campus making things better, faster, cheaper.
Alejandro: What were the typical metrics, for example, like Skype or Facebook, would be more intrigued about learning more?
Peter Bailis: The broader trend we’ve seen, which is interesting is, inside of a modern — any modern organization. Anyone but the very late adopters of technology. People have defined OKRs and KPIs for their business. If it’s marketing, I look at activation, retention, churn, lifetime value. If it’s product, I look at engagement, quality of service. I might look at adoption of new features. If it’s finance, I’m going to look at margin, various measures of efficiency, revenue. It’s almost become ubiquitous within the typical organizations, especially enterprise, to collect and track massive numbers of metrics. Typically, within a given team, you’ll have a weekly standup, and each team would be responsible in tracking five, typically no more than ten metrics that they care about that pertain to their steady-state business and also key compelling events like product launches or new campaigns or so on. What we found was this data was coming from these data warehouses. They always have been stored in data warehouses. But because it’s gotten so much cheaper to collect and store this data because of the rise of the Cloud, in particular, they would attract a lot more data than before. So to answer your question directly, it’s every line of business inside of a modern organization that’s tracking these metrics, and they’re all derived from basic data warehouses like Snowflake.
Alejandro: With Sisu, how do you guys make money?
Peter Bailis: I think it’s interesting. A lot of AIML startups will promise the world. It’s not just startups — just the big players like, “Buy [0:17:25], we’ll automate your data science team.” The reality is from a fundamental statistics perspective, and even from a research perspective, I think it’s still an open question of how and whether you can do this. What we do for Sisu and the core value provided for our customers, we researched, and we subsequently scaled out to a much more powerful backend and scalable interface. We help people understand one simple question: why are my key metrics changing? If I’m a marketing operation lead and I’m watching a metric like new product activations over time, any number of BI tools can tell me how is this metric changing? Activations are down 2% this week versus last week. With Sisu, we’re not trying to replace these dashboarding reporting tools because there’s a ton of them, and they all work well, and they do about the same thing. What we want to do is, we want to base and replace the task of digging deep into the data in understanding what’s driving this metric. Activations are down by 2%, but the reason why it’s down by 2% is that we saw a drop-off in premium subscribers from the mobile channel. We saw an increase in standard subscribers from instore purchases. Breaking down the many thousands to millions of factors behind any individual metric, and making it interpretable for an end-user to understand. Take it for granted — you can search trillions of documents on the internet and get a great answer back for free with services like Google today. But there’s no service today that can do the equivalent or structure data inside a private organization. Our value profits helping people understand once they’ve defined key metrics why are these metrics changing in seconds. And as the data continues to change, keeping up to date as to what’s most important.
Alejandro: Really interesting. To build this up, it requires quite a bit of money. How much capital have you guys raised to date?
Peter Bailis: We just closed a Series B led by NEA, Peter Sonsini, with participation from Andreessen investors from Andreessen Horowitz, Ben Horowitz. Also, the Andreessen Horowitz Cultural Leadership Fund and Green Bay Ventures. That round was 52.5 million. So we raised 66.7 million total.
Alejandro: So 66.7 million total in less than two years. How do you do this, Peter?
Peter Bailis: I think there are two factors. 1) The market for this type of analytics is massive. Everyone wants Enterprise AIML, and no one knows what it looks like. A lot of people are trying it, and there’s a lot of lofty promises and poor execution here. I think we have a pretty unique perspective informed by our work that’s very pragmatic. Customers always ask us, “Can you do time series forecasting? Can you do predictions for me? Can you clean up my data for me?” We say, “No, no, and no. We do one thing incredibly well, which is we’re going to tell you why your metrics are changing. We’re going to be the best in the world at these diagnostic analytics.” I think that a clear perspective, coupled by our early track record, has made raising capital, especially in this environment where there’s massive market demand very attractive. And we have some great people take the bet that we can deliver on this. I think the other thing, too, is why would we raise this money? This is another reason why we have to actually make the decision to go fulltime on this myself is this is a product that I don’t think could be built by just a team of machine-learning researchers, or just a team of database system researchers, or database engineers, or just UI. This is a product experience that requires a combination of world-class execution and machine learning, data engineering, and ViewX and product. To do that, and to attract that type of talent and to scale out and achieve our full potential is a capital-intensive affair. So this capital is letting us take a big swing while iterating very quickly and very diligently and in a focused way with our early userbase.
Alejandro: It seems that Andreessen Horowitz has been a big sponsor, now, when it comes to leading the rounds. I’ve seen Ben Horowitz literally going into labs of universities, based on other founders that I’ve spoken with, and giving like 50 million, just like that, to something that is not really tangible. So how did you guys come across them? Was it Ben Horowitz, or how did you guys get in touch with Andreessen Horowitz?
Peter Bailis: We had some interesting deployments of the research we were doing well in advance of even incorporating the company. This was stuff that we were giving away for free and trying to get people to adopt it. We had some interesting use cases, and online services, and telecom, and some manufacture uses. We had proven out the technology could work, and the big question: What will be the business around this, and how will we reach the maximum number of people? We spent a lot of time with a bunch of friendly folks, largely in the VC space talking to potential customers and understanding what this landscape looks like, and figuring out what would be our unique differentiator, if anything, to go and build a company in this space? So it took a while to get conviction around the space and understand what the structure of the company would look like and what we wanted to raise, and so on. Then as soon as we were ready to go and do that, we had a fast initial fundraise for the Series A and was introed to Ben through one of my mentors and former grad school advisors, Ali Ghodsi, David Riggs.
Alejandro: Oh, yeah.
Peter Bailis: When I met Ben, my philosophy is, I have a lot of opinions and some expertise and data. But at each step along this journey, I want to work with the best possible people who can help complement my strengths and weaknesses. The ability to partner with someone who’s not just a great investor, but has been an operator himself and been in the trenches and understands all of the blood, sweat, and tears behind doing a deadlift of starting a company from scratch. Ben’s a phenomenal partner. The references that I had gotten from my network, everyone from Ali to other folks at Databricks, talking to the folks from Nasira. It was clear that Ben was a fantastic partner from this outset, starting the company with zero lines of code written, zero customers, and huge market potential.
Alejandro: It’s amazing because when I was mentioning that statement earlier — I remember when Ali Ghodsi came to the show as well, he was sharing that story when he was also a researcher. Ben literally saw what they were doing, and he just knew that was going to be really big. So your story reminded me. I thought it was similar. So it’s funny that you mentioned Ali. Really cool. Investors always talk about the market, like if your market is big, that’s also going to either limit for the positive, for the negative, the potential returns that they might get from their investments. How big do you think your market could be?
Peter Bailis: It’s a great question. It’s one we get a lot. To put it bluntly, if you think about what’s going on, just look at Snowflake as an example. Snowflake going up on the right, gearing up for a bunch for mega-rounds, gearing up for IPO, it’s clear that the enterprise use of storage is not slowing down anytime soon. The question I always ask myself is if in ten years the best thing we have to make use of all this structured data — like more data than was online when Google launched, in one of these typical enterprise warehouses. If the best thing we have for making use of this data is Microsoft Excel, which is the number one best-selling application of all time. I won’t say best-selling — largest economic impact. Clearly doesn’t scale to Snowflake scale. Or a BI tool, which is the same form factor that we’ve had since 1995. Or, it’s Python Notebooks, which I think more and more people are coding, and that’s great. But I don’t think everyone in the world should have to code in order to make use of this data. Something’s gone horribly wrong if that’s where we end up in ten years, given the ascendency of this data availability in the enterprise. So I think Microsoft scale opportunity or Google scale, you pick in terms of making use of this data at scale and everyday decision-making inside a business. And I think, realistically, the majority of the world’s businesses will be making data-informed decisions using next-generation data tooling like Sisu in the next ten years. Now, are we the ones that capture that market to enable every business operator to leverage all the data at their fingertips? I don’t know, but I think we have a good shot at making a big dent there, and I think every company — you know, we talk about every company becoming an AI company. I don’t think every company needs AI, but I think to stay competitive and relevant, every company’s going to be able to use data. I think today’s tools — you’re already starting to see this in terms of a lot of the homegrown efforts and a lot of the high-tech companies. They just aren’t up to task. There’s an opportunity for a basic clean slate redesign. That’s what we’re playing for essentially, and that’s also why we’ve raised this capital.
Read More: Drew McElroy On Raising Over $100 Million To Disrupt A $120 Billion Industry
Alejandro: Obviously, as well, for a business of this nature, probably the biggest hurdles might be on creating and developing the sales team because sales for enterprise is a beast. So tell us about how you’re seeing this.
Peter Bailis: One of the things that’s been awesome to work with Ben on, and I think since the early days, he’s been helpful with and a key component of is, one of the key challenges we face in the market is being focused about who we target, who we work with as early customers. If you tell someone, “I’m going to give you a magic box, and we’ll tell you why your key metrics are changing. Do you want this? Do you want to try this?” Everyone’s going to say, “Yes.” It’s like why wouldn’t you want this clear value if it does what it says on the box. From the technology perspective, we have a clear advantage and insight in how to build this, and a great team is going to have to build it. So let’s just say we can do that. How do you prioritize when that’s such a massive market? One of the biggest pieces of emphasis in our early-stage go to market learning to date has been, how do we qualify what a great user looks like, and narrowing our target to find people who are able to adapt very quickly, who have invested in a technology required so that we can get started — do a pilot in an afternoon as opposed to a three-month implementation period. Then, most importantly, can take action on the results we’re giving them. So to give a flavor of what we have narrowed in on, we work people who know exactly who their customers are. A good example is Samsung is one of our public customers. They, obviously, sell phones, and they know what to buy and what’s sold on what carriers and so on. An example of a customer that would not be a great fit right now is Coca Cola. Coca Cola sells to distributors, and then they sell to folks like 7-11; 7-11 sells their products for them. They have no idea who’s buying. So Coca Cola can track how many sales they’ve shipped. They have no idea if it’s people in the mid-west, if it’s repeat customers, what makes those customers who are great customers, actually great. That’s a big part of this. We’re cloud native, so we qualify very hard on people’s willingness to adopt cloud software. My thesis is that in five years, everyone’s going to be in the Cloud, and the ones that aren’t in the Cloud, we’re not going to be the ones to bring them there. Then, I think one of the big ones that I think is overlooked in the space — it’s a super crowded space. Getting insights and getting value from data is totally noisy. The reality is when you talk about insights from data. Insights are bs. If I tell you something is insightful, that doesn’t change your behavior. It’s like if a tree falls in the forest, does it matter? So we try to find people who are able to take action based on the results we show to change their marketing campaigns, change their product strategy, change their business operations. We have a tight way to qualify in and qualify out prospects, and a strong belief that over time, the number of people who qualify is going to go up rather than down.
Alejandro: Got it. One thing that is clear to me is that one of the issues that companies experience, and especially early-stage companies that could be fatal, is that for the most part, they make decisions based out of assumptions. I think that right now, what you guys are doing is removing that completely. Is that right?
Peter Bailis: Do you mean for our customers or ourselves?
Alejandro: For your customers now, you’re literally now able to show them what’s happening.
Peter Bailis: Yeah. It’s kind of funny. I think you’re absolutely right. In a nutshell, data-driven decision-making is not in a new idea. So people making data-driven decisions beginning with modern accounting, you just had profit and loss as your two indicators, and they’re lagging in terms of indicators. The type of customers that we work with and that we usually work with have already made the decision to track leading indicators like engagement and activation and margins, and so on. But they lack — not the aptitude, but the resources and the time to go and dig into all their data and figure out what is my data telling me about how I’m performing operationally? The sad part of this is, as businesses enter into more and more competitive environments and as data continues to update over time, the answer to what is most important and what’s working well and what’s not working well, to do more of what’s working well, and to change what’s not working well, it’s constantly changing. The value add, and the promise for customers, especially the ones who are particularly successful on the platform is exactly what you said. I’m already doing a bunch of stuff on a daily basis or a weekly basis in terms of, say, how I’m running my marketing team. But if I can be 10% more informed by knowing exactly what has changed in the fundamentals of my marketing pipeline over the last 12 hours, the last 24 hours, I’m going to be that much more informed in order to make these decisions. Computer science has only been around for 50 years, data, at this scale only been around for the last 15 to 20 years, depending on which organization you’re talking with. It makes sense that we have this decision-making process, which is based on gut instinct and more or less which side of the bed we woke up on. In many cases, gut instinct is valuable. We don’t want to replace that. I think that’s one of the fallacies with a lot of AI and ML products are like, you don’t need your humans anymore. You’re going to show up the market teams in the Cloud. We think of this type of analytics as a way to augment human decision-making and check some of those assumptions and biases and beliefs using the data in real-time.
Alejandro: Very cool. One of the questions that I typically ask the folks that we have on the show is that — for you, this year has been unbelievable. What a rocket ship of a ride, Peter! Obviously, it has probably come with a lot of different lessons and learnings because when you’re building things at this type of hypergrowth, the learning and the speed at which you learn is remarkable. Now, if you had the opportunity to go back in time and still when you were perhaps at that point where you were about to make the decision of going on a leave from Stanford and launching Sisu, what would be that one piece of business advice that you would give to your younger self, and why, knowing what you know now?
Peter Bailis: That’s a good question. I think that one of the things we’ve done a good job of is keeping a really high bar and building out an amazing team where we’ve brought together different disciplines. Amechee as our second higher she basically joined from the Twitter timeline team, where she lets some of the UX redesign on the inner-border tweets to outer-border tweets, and pairing her together with something like Vlad who was the top PhD program in the country at Berkeley machine learning. He had him drop out, put them in the room together at early stage. I would definitely do that again. I think the thing I would try to do more of is, focus even more on hiring. We’re pretty far along for less than 18 months in as a company, but I would love to find even more Vlads and Amechees to work with. In some sense, we’re at 25 now and growing quite a bit. But almost investing even more in terms of talent, even getting ahead of talent, which we’re hiring for now, but giving one even as one of the first five hires would be fantastic. So I think when I talk with Ben a lot is about people, product, profits in that order. I feel so fortunate. One of the things I enjoyed relative to being on campus, I loved getting to know and work with people who are truly masters of their craft in areas other than core CS. I feel like hiring — you can, obviously, take this to an extreme and over-hire and have a bunch of problems with this, but I think investing even more into hiring and scaling the team faster would have been great. So maybe if I went back and played that, I’d be back on the show today in saying, “I wish I hadn’t hired that fast because there are a lot of special parts of culture that take time to build and develop,” but I do feel like, especially now, with so much opportunity in front of us, it’s always going to be a prioritization problem, but I do feel like we probably could have hired a little bit quicker. The other thing I’d probably do is spend more time with my wife because there’s always more stuff to do, and there are only so many weekends to spend time with your loved ones.
Alejandro: I hear you. So, really cool, Peter. For the folks that are listening, what is the best way for them to reach out and say hi?
Peter Bailis: I love to chat. My email is [email protected] – you can signup for a trial on our website. Take a look at sisu.ai, and I would love to get feedback. If you’re interested, I’ll try to figure out why your metrics are changing. We’re really excited to talk to people, especially folks who are within their own organizations. Frequently, we see some of the folks who are most successful partners with us. They’re inside the organization. They realize they have this massive store of data at their disposal, and there are almost changes within these organizations trying to change the way their companies are using, working with, thinking about data. So if this is resonating, I would love to follow-up and hear where you’re using data effectively, where you’d like to use it more effectively, and see if Sisu can help.
Alejandro: Amazing. Well, Peter, thank you so much for being on the DealMakers show today.
Peter Bailis: Thank you so much for having me. I really enjoy the show, and I appreciate your time and questions. It’s been a lot of fun.
* * *
If you like the show, make sure that you hit that subscribe button. If you can leave a review as well, that would be fantastic. And if you got any value either from this episode or from the show itself, share it with a friend. Perhaps they will also appreciate it. Also, remember, if you need any help, whether it is with your fundraising efforts or with selling your business, you can reach me at [email protected].
Podcast: Play in new window | Download
Subscribe: Apple Podcasts | Google Podcasts | Spotify | Stitcher | TuneIn | RSS | More
Facebook Comments